The robot apocalypse has been delayed until further notice
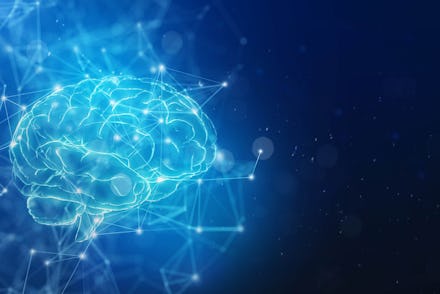
It may seem like artificial intelligence is quickly seeping into just about everything. While that might raise concerns about a Skynet-style takeover, the quiet secret about AI is that it isn't taking over. In fact, some experts believe that AI in its current form is starting to slow down, reaching its maximum capacity — at least for the time being. In an interview with Wired, Facebook's head of AI, Jerome Pesenti, theorized that the development of artificial intelligence and machine learning is about to "hit the wall."
According to Pesenti, the deep learning mechanisms that currently help power and push the advancement of AI are pushing up against their limitations. Some of that has to do with the lack of necessary computing power to continue improving. He told Wired that deep learning works best when it can be scaled up and given more room to operate. Unfortunately, doing that is becoming cost-prohibitive and large-scale projects are becoming next to impossible to conduct. "The rate of progress is not sustainable," Presenti said. "If you look at top experiments, each year the cost it going up 10-fold. Right now, an experiment might be in seven figures, but it’s not going to go to nine or 10 figures, it’s not possible, nobody can afford that."
There is a concept in computing known as Moore's Law, which posits that computing power doubles every two years. In the case of AI development, according to a recent study published by OpenAI, the amount of computing power used in AI training has doubled every 3.4 months, a massive acceleration to the standard progression we are used to. Because of that speed of advancement, OpenAI believes that AI has required a 300,000-times increase in computing power since 2012, as opposed to the seven-times increase that it would typically get under Moore's law. That trend is not sustainable. Limitations to the development of processing power is already starting to slow the progress of AI and machine learning, especially since research shows that the one thing that leads to predictably better performance from AI systems is access to more computing power. There is also theoretically a cap on just how advanced an AI can become because of actual limitations to computing capabilities. OpenAI noted that at some point, simple physics will limit the potential efficiency of chips that are used to power and train AI systems.
Quantum computing represents the best chance to break through some of those limitations, and significant gains are being made in that field. Earlier this year, Google announced that it had achieved "quantum supremacy," developing a quantum processor capable of solving computations that would take a standard computer more than 10,000 years to complete — though the claim has been called into question. This week, Amazon announced a plan to offer quantum computing as a service, similar to how its Amazon Web Services offers servers to companies so they don't need to build and host their own. These could represent points of potential progress for AI, which requires increasing access to computing power to continue the rapid progress it has made in a short period of time.
Beyond the inability to keep up with the exponentially increasing demand for computing power that AI requires, there are also limitations in how AI is trained in the first place. While Presenti noted to Wired that "You can apply deep learning to mathematics, to understanding proteins, there are so many things you can do with it," he also acknowledged that "Deep learning and current AI, if you are really honest, has a lot of limitations." He noted that even with the significant jumps forward over the last decade or so, AI and machine learning are still a long way from being able to duplicate human intelligence. These systems do not have the sense to think for themselves or account for little intricacies the way the human brain can.
The one "human" thing that AI is very good at replicating is our biases. When training artificial intelligence, researchers typically feed the systems large sets of data that they use to process and learn from. Unfortunately, many of those datasets are riddled with examples of human flaws and preconceived biases that they may not even realize are informing the work. Laura Douglas, an AI researcher and CEO of myLevels, once warned that when we teach machines on information that already contains our own misconceptions, the algorithms have a tendency of amplifying those biases rather than correcting for them. We see these failures in action constantly within existing AI systems. Earlier this year, it was reported that school districts across the country are using AI systems that are unfairly punishing people of color and disadvantaged students for mistakes that humans could more accurately interpret and process. In 2015, Pro Publica showed instances of automated sentencing systems displaying racial bias by falsely suggesting a higher rate of recidivism for black defendants while predicting a far lower rate of recidivism for white defendants. Similarly, a study found that predictive crime tools have a tendency to disproportionately push police into minority neighborhoods even when crime statistics in the area don't reflect the need for more policing. Until these data sets and the collection process is improved and stripped of human biases, AI will likely continue to perpetuate and even exacerbate these flaws, limiting the capability to learn and improve.
AI as we know it may be reaching its ceiling. While it still has room to grow in some areas, the science fiction future in which machines learn and improve on the fly, performing human-like thinking and processing tasks, is probably not in our near-term future. Professor Michael Wooldridge, the head of the Department of Computer Science at Hertford College, has warned that "there is, clearly, an AI bubble at present." That seems to be borne out by most indicators. The Artificial Intelligence Index releases a report every year that takes into account the progress made in AI year-over-year. It has found that even with breakthroughs happening on the regular, progress is considerably slower than we may have been led to believe. AI systems are getting better at certain, specific tasks; often ones with a specific, contained goal, like playing a game of Go or processing images. But we are still a long way from the singularity or any form of "artificial general intelligence" in which machines become smart enough to understand and process information in the same way a human can. Accomplishing that, assuming it is something that we should want to accomplish, will require significant advances in computing power and major improvements to how we currently train and teach AI. Pending that, consider yourself safe from any sort of robot apocalypse for the time being.