Scientists are trying to make robots more trustworthy
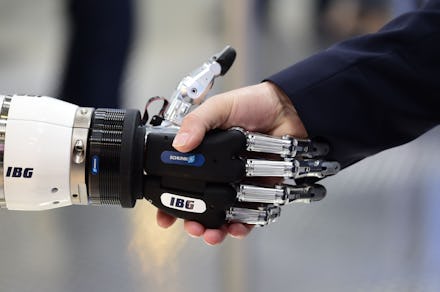
Artificial intelligence is entering our lives in many ways – on our smartphones, in our homes, in our cars. These systems can help people make appointments, drive and even diagnose illnesses. But as AI systems continue to serve important and collaborative roles in people’s lives, a natural question is: Can I trust them? How do I know they will do what I expect?
Explainable AI (XAI) is a branch of AI research that examines how artificial agents can be made more transparent and trustworthy to their human users. Trustworthiness is essential if robots and people are to work together. XAI seeks to develop AI systems that human beings find trustworthy – while also performing well to fulfill designed tasks.
At the Center for Vision, Cognition, Learning, and Autonomy at UCLA, we and our colleagues are interested in what factors make machines more trustworthy, and how well different learning algorithms enable trust. Our lab uses a type of knowledge representation – a model of the world that an AI uses to interpret its surroundings and make decisions – that can be more easily understood by humans. This naturally aids in explanation and transparency, thereby improving trust of human users.
In our latest research, we experimented with different ways a robot could explain its actions to a human observer. Interestingly, the forms of explanation that fostered the most human trust did not correspond to the learning algorithms that produced the best task performance. This suggests performance and explanation are not inherently dependent upon each other – optimizing for one alone may not lead to the best outcome for the other. This divergence calls for robot designs that takes into account both good task performance and trustworthy explanations.
Teaching robots
In undertaking this study, our group was interested in two things. How does a robot best learn to perform a particular task? Then, how do people respond to the robot’s explanation of its actions?
We taught a robot to learn from human demonstrations how to open a medicine bottle with a safety lock. A person wore a tactile glove that recorded the poses and forces of the human hand as it opened the bottle. That information helped the robot learn what the human did in two ways: symbolic and haptic. Symbolic refers to meaningful representations of your actions: for example, the word “grasp.” Haptic refers to the feelings associated with your body’s postures and motions: for example, the sensation of your fingers closing together.
First, the robot learned a symbolic model that encodes the sequence of steps needed to complete the task of opening the bottle. Second, the robot learned a haptic model that allows the robot to “imagine” itself in the role of the human demonstrator and predict what action a person would take when encountering particular poses and forces.
It turns out the robot was able to achieve its best performance when combining the symbolic and haptic components. The robot did better using knowledge of the steps for performing the task and real-time sensing from its gripper than using either alone.
Gaining human trust
Now that the robot knows what to do, how can it explain its behavior to a person? And how well does that explanation foster human trust?
To explain its actions, the robot can draw on its internal decision process as well as its behavior. The symbolic model provides step-by-step descriptions of the robot’s actions, and the haptic model provides a sense of what the robot gripper is “feeling.”
In our experiment, we added an additional explanation for humans: a text write-up that provided a summary after the robot has finished attempting to open the medicine bottle. We wanted to see if summary descriptions would be as effective as the step-by-step symbolic explanation to gain human trust.
We asked 150 human participants, divided into four groups, to observe the robot attempting to open the medicine bottle. The robot then gave each group a different explanation of the task: symbolic, step-by-step, haptic – arm positions and motions, text summary, or symbolic and haptic together. A baseline group observed only a video of the robot attempting to open the bottle, without providing any additional explanations.
We found that providing both the symbolic and haptic explanations fostered the most trust, with the symbolic component contributing the most. Interestingly, the explanation in the form of a text summary didn’t foster more trust than simply watching the robot perform the task, indicating that humans prefer robots to give step-by-step explanations of what they’re doing.
Designing for both performance and trust
The most interesting outcome of this research is that what makes robots perform well is not the same as what makes people see them as trustworthy. The robot needed both the symbolic and haptic components to do the best job. But it was the symbolic explanation that made people trust the robot most.
This divergence highlights important goals for future AI and robotics research: to focus on pursuing both task performance and explainability. Only focusing on task performance may not lead to a robot that explains itself well. Our lab uses a hybrid model to provide both high performance and trustworthy explanations.
Performance and explanation do not naturally complement each other, so both goals need to be a priority from the start when building AI systems. This work represents an important step in systematically studying how human-machine relationships develop, but much more needs to be done. A challenging step for future research will be to move from “I trust the robot to do X” to “I trust the robot.”
For robots to earn a place in people’s daily lives, humans need to trust their robotic counterparts. Understanding how robots can provide explanations that foster human trust is an important step toward enabling humans and robots to work together.
This article was originally published on The Conversation by Mark Edmonds and Yixin Zhu. Read the original article here.